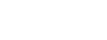
The In recent years, Metals and mining organizations across the world has started to follow in the footsteps of other industry players like Retail and Consumer to embark on their digital transformation journeys spanning across the entire value chain – from raw material sourcing to manufacturing, distribution and overall ecosystem development. In this two-part series on "Digital Transformation in Metals and Mining industry" we cover some of the interesting use cases of digital transformation across the entire value chain.
In the first part of the series we cover some interesting use cases in the upstream value chain
DIGITAL TRANSFORMATION USE-CASES IN RAW MATERIAL SOURCING
DIGITAL TRANSFORMATION USE-CASES IN MANUFACTURING